Emmanuel Dufourq and Lorène Jeantet collaborated with an international team of scientists from the City University of New York, the University of Antananarivo and Centre ValBio in Madagascar, where they leveraged the power of deep learning to transform an approach to primate conservation. Through the innovative use of passive acoustic monitoring (PAM) paired with machine learning, the team has not only streamlined the process of studying the critically endangered black-and-white ruffed lemur in Madagascar, but have also unveiled the first quantitative, published evidence of their nocturnal vocal activities. These lemurs are one of the world’s most endangered mammals. The study took place in Ranomafana National Park, Madagascar over several months. The figure below provides a spectrogram, a snapshot of sound, which reveals the vocalisations of the ruffed lemurs. The machine learning models were tasked to find these vocalisation events within over a terabyte of audio data.
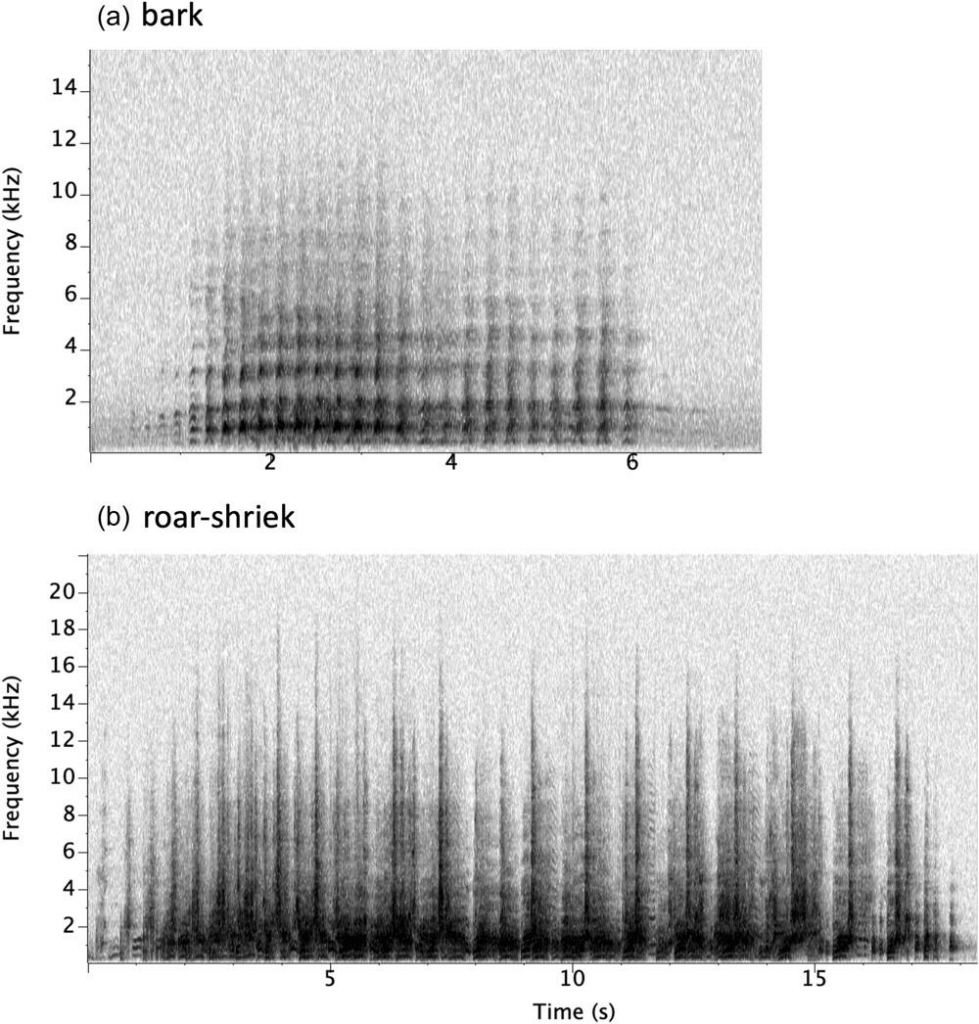
While PAM and automated detection models have long been utilized in marine mammal research, their application in studying nonflying terrestrial mammals, especially in primatology, remains relatively underexplored. This is particularly striking given Madagascar’s rich biodiversity and the alarming rate at which it has lost half of its forests over the past 60 years, making the need for innovative conservation methods like PAM more critical than ever.
This research demonstrates the efficacy of PAM and machine learning over traditional methods, offering a solution that is not only cost-effective but also scalable, requiring significantly less labor and time. By combining simultaneous PAM and focal follows, this study not only compares these methods but also highlights the undeniable benefits of integrating technology with conservation efforts. The team collected 2,300 hours of audio data which was processed at AIMS, and jointly analysed with researchers at the City University of New York. Jointly, the team from AIMS produced open-source easy-to-use software to decrease the barrier to entry for PAM and machine learning. Link to publication https://onlinelibrary.wiley.com/doi/10.1002/ajp.23599